EO4EU Shows Artificial Intelligence and Machine Learning Potential Impacts for Satellite Data
The EO4EU project aims to improve access to and use of Earth Observation (EO) data for a wide range of users, from professionals to the general public, through the use of innovative tools and technologies such as Artificial Intelligent (AI) modules, augmented reality and advance visualization.
This project comes at an opportune time for its development. In recent years, AI and Machine Learning (ML) have played a central role in the analysis and management of data from EO satellites, processing and interpreting large volumes of data, and becoming increasingly indispensable tools to meet the challenges posed by the complexity of the environments in which we live.
These technologies automate complex processes and turn large amounts of information into valuable insights. This unlocks the potential of EO data, allowing for more efficient and accurate monitoring of the planet, the detection of hidden patterns, and better information management.
Among the key innovations, the project introduces ML methods for semantic annotation to improve the quality and interpretability of existing and future data: this approach allows large amounts of information to be automatically labeled, making it more consistent and accessible for a wide range of applications, from scientific research to environmental management.
Therefore, as it is easy to guess, AI and ML technologies find applications in different EO domains and topics, as it can be observed also from the seven use cases (UCs) integrated in the EO4EU platform. These use cases show how AI and ML are not just tools for technological innovation, but real solutions to global challenges, from resource optimisation to natural disaster prevention.
In the Ocean Monitoring and Optimised Routes UC, for example, EO technologies are combined with data collected from ship sensors to optimize sea routes. The aim is to reduce fuel consumption and improve safety, using routing algorithms such as A* and Dijkstra together with Reinforcement Learning to create an innovative weather routing module.
The Food Security and Crop Forecasting UC makes use of a deep learning model, the MLPClassifier, to predict the future yield of key crops for food security. Based on CMCC climate scenarios, the model identifies areas where crops may be sustainable in the future, supporting strategic agricultural decisions.
In the Climate Impacts on Forest Ecosystems UC AI models, such as Boosted Regression Tree, Random Forest and MARS are used to study how climate change affects forests. These models help to understand and predict the adaptation or migration of forest species to areas with more favorable climatic conditions.
The Soil Erosion Prevention UC uses an innovative approach, an improved land cover map through self-supervised classification, to more accurately estimate the C-factor, a crucial parameter for erosion assessment. Temporal downscaling ML techniques also make it possible to predict the intensity of rainfall events at 30-minute intervals, improving the estimation of erosion risk at the event level.
In the Environmental Pest Monitoring UC AI is used to predict and monitor locust invasions by combining climatic and terrestrial observation data. By analyzing various environmental and temporal factors, the model identifies areas at risk of locust breeding, helping to monitor the distribution of these pests and implement mitigation actions.
Finally, the Civil Protection and Fire Management UC makes use of AI to improve emergency management by producing daily fire risk bulletins, identifying the areas most at risk at the municipal level. The technology allows the integration of static and dynamic data, thus supporting civil protection in planning and responding to fires, earthquakes and landslides.
Conclusion
The different EO4EU case studies highlight how AI and ML have become indispensable tools for scientific research and the efficient use of EO data. These technologies, in their different approaches, have the potential to radically transform the way we manage natural resources and address global climate challenges, improving our ability to monitor and protect the environment.
However, they are very complex technologies that require specific knowledge and skills. Integrating AI algorithms into a simple system that is accessible to all users is therefore crucial, and the EO4EU platform aims to facilitate this process. Thanks to AI and advanced data visualization and analysis tools, EO4EU makes the use of these complex technologies simple and intuitive, allowing a wide range of users, from professionals to ordinary users, to exploit the full potential of EO data for more informed decisions and concrete actions in protecting the planet.
Contribution: MEEO
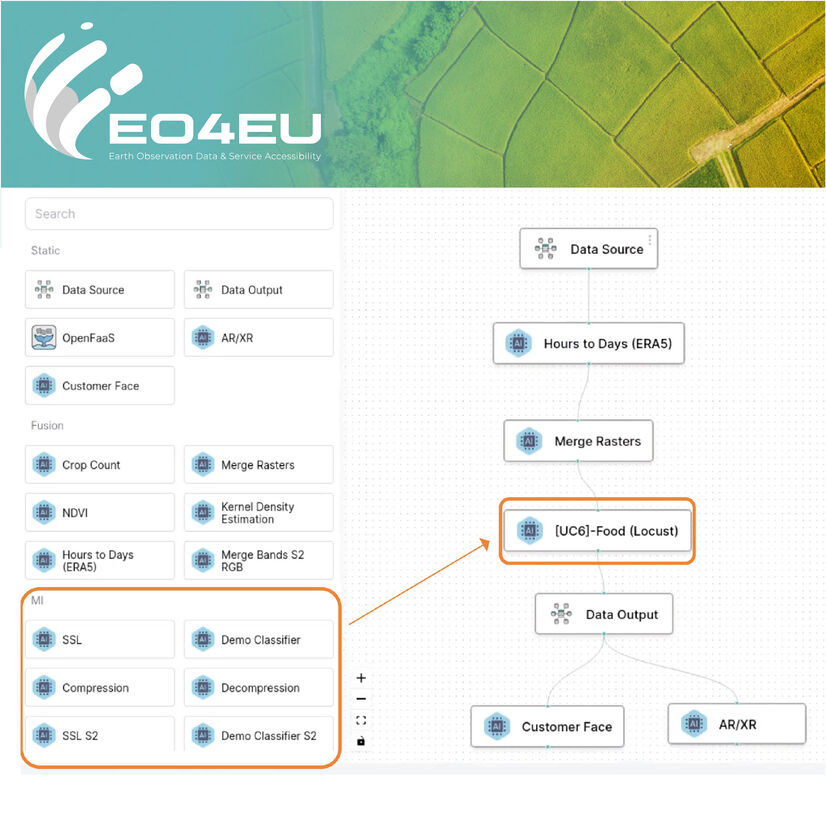