Developing Earth Observation Knowledge Graphs
In a world overflowing with data, particularly in the realm of earth observations, extracting useful insights poses a serious challenge. Knowledge Graphs (KGs) have emerged as a revolutionary technology, providing an organized, interconnected framework essential for harnessing complex data sets. This structured approach is especially valuable for synthesizing data related to earth's environmental and climatic phenomena, enabling clearer insights and more informed decision-making. Developing a KG for earth observation is a collaborative venture that involves numerous stakeholders, including data scientists, policymakers, and domain experts across Europe. This process is not static; it evolves with emerging data sources, new research findings, and updated policy requirements.
Integrating Diverse Data Sources
The conversion of diverse data sets into a cohesive KG begins with the tasks of data acquisition and integration. The Copernicus Program exemplifies the EU's commitment to open-access earth observation data, providing essential information such as satellite imagery and atmospheric measurements. Complementary to this, the European Space Agency contributes data from its satellite missions, while the European Environmental Agency offers insights on air quality, biodiversity among others. Collecting and integrating these datasets ensures a useful and comprehensive knowledge framework.
A pivotal step in KG development is data enrichment, which involves refining the semantic quality of the graph. This includes entity disambiguation—resolving ambiguities to ensure accuracy—and linking information to existing ontologies or external knowledge bases. Semantic enrichment transforms raw data into usable, insightful knowledge, enhancing analysis capabilities and fostering improved decision-making.
Designing and Structuring the Knowledge Graph
The foundation of an effective KG lies in its schema and ontology design, which involves representing entities and their relationships graphically. Using standards like RDF Schema and OWL ensures that the data remains interoperable and consistent across diverse platforms. For instance, the Semantic Sensor Network Ontology provides a framework for describing sensors within the KG, while resources such as the NASA Earth Observation Ontology standardize descriptions of Earth Science data, ensuring seamless integration.
Achieving data consistency requires careful transformation and cleaning processes. Data formats must be standardized, errors corrected, and duplicates removed to ensure high quality and compatibility with the KG's schema. Once processed, data undergo extraction and semantic enrichment, where entities are identified and linked, enhancing the graph's detail and utility.
Extracting and Linking Relationships
Relationship extraction and linking are crucial steps in building KGs as they involve identifying and capturing the relationships between entities and establishing connections within the graph. This process involves analyzing data sources, such as text documents or structured data, to extract meaningful relationships that represent the semantic connections between entities. Natural Language Processing (NLP) and machine learning techniques, including dependency parsing, pattern matching, and information extraction, are utilized to identify these relationships. Dependency parsing algorithms analyze the grammatical structure of sentences to identify syntactic relationships,
while pattern matching techniques capture specific linguistic patterns that indicate relationships.
Information extraction techniques further identify key phrases and attributes to determine relationships from unstructured or semi-structured data sources. Relationship linking involves finding and matching the semantic relationships between entities in natural language text to the corresponding relationships in the KG. This step is essential for enabling natural language understanding and interaction with the graph, such as question answering and text summarization. It involves mapping and aligning entity mentions within the text to the appropriate entities in the graph, often leveraging entity disambiguation techniques and external knowledge bases. Labels or types are assigned to the extracted relationships to indicate their nature or semantics, and connections are established between entities in the KG by creating edges that represent these relationships. These edges enable efficient navigation and querying within the KG, enhancing its semantic richness and interconnectivity, and facilitating more advanced analysis,
reasoning, and knowledge discovery.
Knowledge Graphs are redefining how we approach earth observation data, transforming disparate datasets into a unified, insightful framework. As the EU continues to lead in earth observation initiatives, the implementation of KGs will ensure adaptability, efficiency, and enhanced decision-making, ultimately contributing to sustainable environmental management and policy development.
Contribution: Novelcore
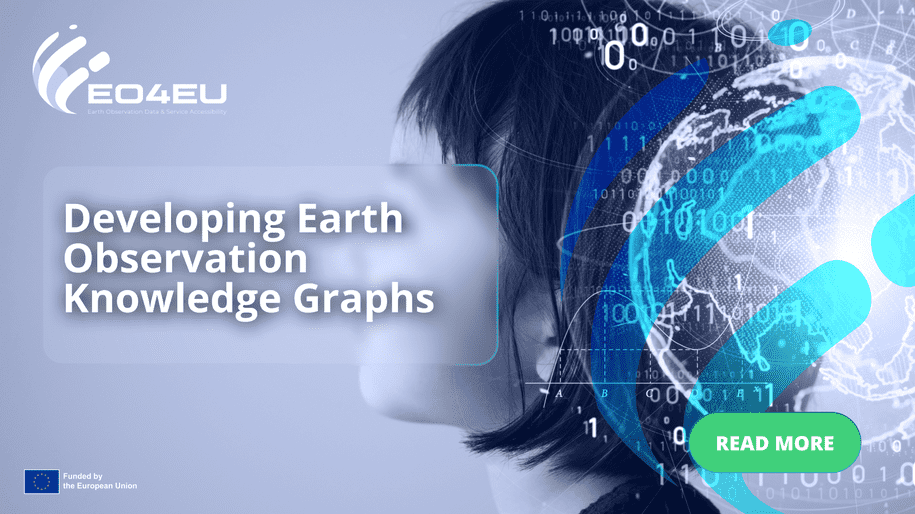