The use case proposed for this project is an information service of locust plague impact assessment and prediction. Based on a preliminary activity that originally involved an NGO currently operating in Yemen, SISTEMA is currently working on improving the damage assessment solution extending it with a predictive solution for preventive actions, to provide the NGO community and the international institutions with a more robust service.
Desert Locust plague is strongly influenced by climate conditions and vegetation status. So far, all the studies aimed at describing and monitoring this phenomenon have approached separately the Earth Observation techniques and Climate predictions analysis. In the prototype service proposed by SISTEMA, EO and Climate data are processed together by means of AI/ML techniques to obtain a more reliable and effective monitoring and prediction service. The scope of this use case is the evolution of the information service by introducing conceptual improvements with new climate models for prediction and technical improvements with the exploitation of HPC infrastructure for the computational aspects. The partners of this use case are CMCC for what concerns their expertise both in the climate models domain and in the configuration and optimization of HPC resources, and CINECA for what concerns the computational infrastructure exploitation.
Challenge
The Desert Locust (Schistocerca gregaria), found in various parts of Africa, Asia, and the Middle East, is considered the most destructive migratory pest in the world because it is highly mobile and can form swarms containing millions of locusts, leading to devastating impacts on crops, pasture, and fodder. A small swarm (1 km2) can be made up of 80 million locusts and can consume the same amount of food in one day as 35,000 people, while a large swarm can eat up to 1.8 million metric tons of green vegetation, equivalent to enough food to feed 81 million people. Without broad scale control, conservative estimates for locust-related losses including staple crops, livestock production and asset damages are estimated at US$8.5 billion for countries in the wider East Africa region, Djibouti and Yemen. In 2021, the Director-General of the Food and Agriculture Organization of the United Nations (FAO), QU Dongyu, has welcomed an €8 million contribution from the European Union (EU) that will enable countries in the Horn of Africa to maintain a state of readiness in the face of a possible resurgence of Desert Locusts.
To improve this kind of services, the contribution of climate forecast is fundamental to increase the reliability of climate impact prediction and to provide reliable risk information to local operators and to government institutions.
Solution
The monitoring of the desert locust pest can be integrated in two types of answer to such crisis:
- Early warning, to prevent the up surging of the locusts: this answer is mostly preventive by identifying areas that shows good environmental conditions for the locust breeding.
- Situational, to follow the crisis already going on, identifying the swarms and their impact to provide an efficient humanitarian answer.
In the context of Earth Observation, such work can be performed, indeed model and sensors are providing meaningful information at every stage concerning the ecosystem of the locust. From the precipitation situation to the vegetation development, datasets as the one provided by ERA5 land, can be used for such activity.
Moreover, the FAO is providing a quite complete monitoring of the locust, via the desert locust watch areas that can be easily located and analyzed to identify patterns for a preventive answer of the crisis.
In the context of machine learning, computer vision methods such as image segmentation and / or temporal analysis could be used in such work. Indeed, with the data mentioned above, it exists a possibility of machine learning pipeline to perform a preventive monitoring of the desert locust pest.
The improvements proposed in this use case cover both i) the change detection algorithms to recognize the effect of the presence of locust swarms in agricultural areas and ii) the use of prediction algorithms to identify areas favorable to the presence of locust swarms.
i) A change detection algorithm based on Sentinel 1 and Sentinel 2 data for land cover change detection feature extraction will be used as damage assessment tool. The change detection maps will be then correlated to climate parameters and assimilated into climate models to predict migration, dispersion, and evolution of the pest.
ii) The prediction algorithm will be based on the use of bioclimate model and climate conditions that can be correlated to the presence and the spread of swarms.
Thanks to the proposed solutions, this use case aims to reduce the technological gaps of the currently available services, reaching a larger processing capability with a better reliability of the information content. Moreover, this use case is an excellent setting for incorporating the advantages provided by the self-supervised task.
Current status
At the current stage, data collection and data preparation tasks have been performed. The following datasets have been collected over Africa and south-west Asia:
- Copernicus ERA5 Land data (2m temperature relative humidity)
- NASA GPM IMERG precipitation data
- Soil Moisture data from SMOS
- Vegetation tenure from MODIS
- Copernicus global land cover classification
- FAO Locust watch database
Whenever possible, the datasets have been spatially and temporally homogenized to support model training and validation.
A preliminary model based on a convolutional Long Short-Term Memory (ConvLSTM) model has been already implemented. Sensitivity analysis to the different parametrizations is currently on going.
This use case aims to reduce the technological gaps of the service, reaching a larger processing capability with a better reliability of the information content.
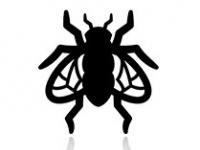
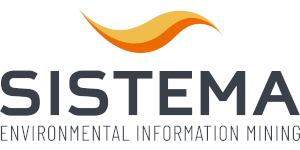
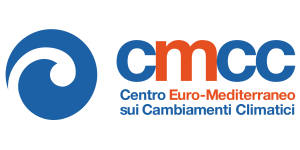