Countries are required to report GHG emissions and removals from land use, land use change, and forestry (LULUCF) under the United Nations Framework Convention on Climate Change (UNFCCC) and the Paris Agreement. Forests act as carbon sinks, absorbing CO₂ from the atmosphere and storing it in biomass, soils, and dead organic matter. Improved forest mapping is crucial for accurately estimating carbon stocks and their changes over time. Without detailed and accurate forest maps, it is difficult to quantify the carbon sequestered by forests or released through deforestation and forest degradation. This accuracy is key for GHG inventories to reflect true emissions and removals. Improved mapping technologies, such as satellite imagery, drones, and remote sensing, allow for better detection of changes in forest cover and forest degradation. Early detection enables corrective measures and ensures that GHG emissions from deforestation are properly accounted for in national inventories.
Challenge
Across the EU, different countries and institutions may adopt different criteria for what constitutes a "forest". These definitions differ based on criteria like minimum canopy cover, area size, tree height, and how land use and land cover are classified. For instance, what qualifies as a forest in one country may not meet the thresholds in another, creating disparities in reported forest areas. Further complicating matters, monitoring mechanisms vary widely across Member States. Some rely on advanced satellite data and remote sensing platforms, while others depend on less frequent National Forest Inventories (NFIs) or use differing analytical methods for interpreting forest data. These variations result in inconsistent tracking of forest cover, deforestation, and degradation, making it difficult to standardize reporting under the LULUCF sector for GHG inventories. While the Copernicus satellite program provides valuable data, its snapshots are limited to specific reference years, falling short of the continuous monitoring needed to capture annual changes in forest cover. To ensure accurate and consistent GHG inventories, the EU must harmonize forest definitions and mapping methods, enhance the integration of remote sensing technologies, and develop validated, precise forest cover maps that can be standardized across all countries.
Solution
The Forest Ecosystems Use Case (UC4) is designed to generate high-resolution forest cover maps using an advanced machine learning model trained on Earth Observation data. Unlike current annual forest cover maps, this service will offer enhanced classification capabilities, distinguishing forest classes from other land cover types, including agricultural and agroforestry areas. Using timeseries analysis, the machine learning model can identify the structural features of each land cover class, based on phenological characteristics and structural attributes associated with different types of vegetation. The model is built on precise ground data sourced from the LUCAS (Land Use/Cover Area Frame Survey), which collects detailed information across Europe on tree height, land cover types, and species composition. This high-quality ground data significantly enhances the robustness and accuracy of the model, ensuring reliable classification of forest cover and supporting better management and conservation efforts.
Current status
A prototype of the machine learning model for UC4 has already been developed, achieving 80% accuracy in forest cover classification. The model is trained using inputs from over 8,000 in-situ data points across Austria, selected as the pilot region due to its significant forest coverage. Key inputs also come from Sentinel-1 data, which provides structural information on vegetation, enhancing the model's ability to distinguish between forest types and other land cover categories. The upcoming integration with the EO4EU platform allows the model to be applied to any user-selected region and year.
Input data
Input data is derived from Sentinel-1 GRD IW, VH band. Data is radiometrically terrain corrected linear gamma0 with Copernicus30m DEM. Sentinel-1 data is retrieved as monthly maxima for each pixel, with a total of 12 images retrieved per selected year.
Impact achieved thanks to the EO4EU Platform
Leveraging Copernicus remote sensing data from the Sentinel mission, which provides high-resolution and frequent (every 5-6 days) measurements, UC4 enables continuous, wall-to-wall mapping of forest ecosystems across Europe. The integration of LUCAS training data ensures standardized forest classification. Indeed, the LUCAS ‘Woodland’ land cover class equals the standard FAO-FRA Forest definition class, providing a consistent basis for reporting. Moreover, the share of forest area derived from LUCAS serves as a key indicator for the Sustainable Development Goal (SDG) 15, which focuses on life on land. The resulting forest maps are crucial not only for accurate national GHG inventories but also for informing essential applications in forest management policies, biodiversity conservation, and land-use regulations. National and local forestry agencies will depend on these maps to effectively manage forest resources, plan reforestation and afforestation initiatives, monitor illegal logging, and promote sustainable forest practices.
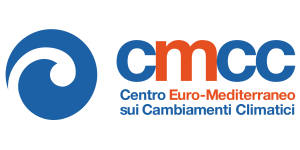
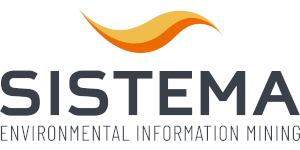
Use case 4, dealing with forest ecosystems, will utilize a variable pallet of input data mostly derived from EO and/or other thematic geo datasets (e.g. from Copernicus services), to exploit forest modelling approaches in the digitalization of the forestry sector, also in line with activities recently undertaken by the collaboration of partners with forestry stakeholders in several EU projects (MADAMES-AX, DESIRA)